The process of coding text responses in surveys and other data collection efforts is labor-intensive for researchers. Manually reviewing each entry and applying relevant codes from a taxonomy is tedious and time-consuming. Existing software options further complicate the process through inefficient interfaces, lack of deduplication, and inflexibility in updating assigned codes.
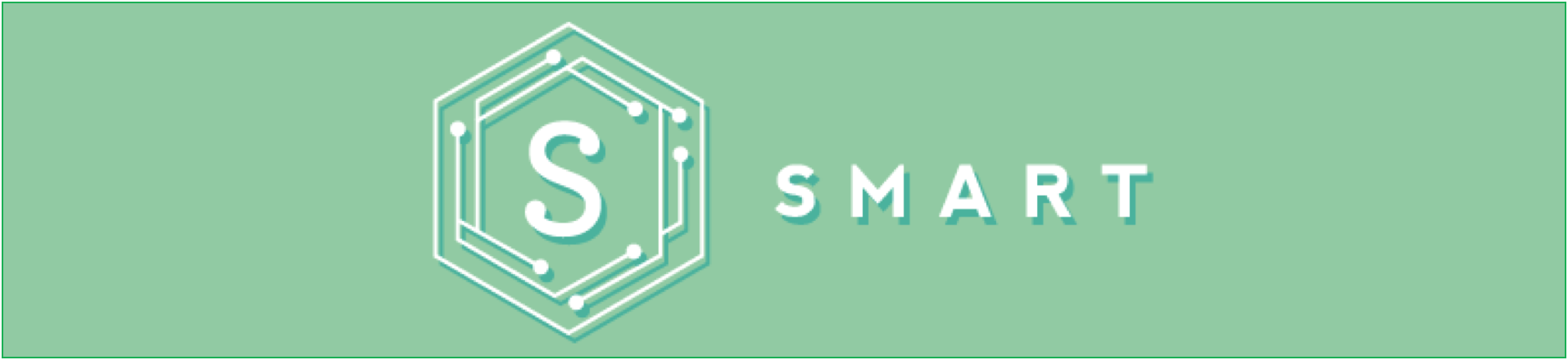
Evolution of SMART: From Machine Learning Labeling to Advanced Survey Coding
SMART—an open-source application originally developed by RTI International in 2018 to assist in labeling data for machine learning models—has evolved into a powerful platform tailored to address common pain points associated with survey coding. SMART employs modern natural language processing techniques to recommend relevant codes interactively as labelers review each text response. This Artificial Intelligence (AI)-assisted label recommendation system helps increase efficiency, reduce manual effort, and improve onboarding for new coding staff—especially for surveys with large, nuanced code taxonomies that contain hundreds or thousands of categories.
Key Features of SMART for Efficient Survey Coding
In addition to label recommendations, SMART provides a robust set of features tailored to survey coding workflows, including the following:
Classical text processing methods like deduplication to reduce coders’ time spent relabeling identical entries
An intuitive management interface that enables project leaders to create, configure, and monitor the progress of multi-coder annotation efforts easily
Comprehensive inter-rater reliability metrics to enable rigorous quality assurance by identifying inconsistencies between human coders
Project-specific label recommendation models can also be trained to provide even more accurate suggestions tailored to each survey’s unique taxonomy. This feature was used on a large education survey, and the accuracy rating improved from 43% to approximately 70%. Through real-world coding use cases, SMART is continually updated and optimized to address challenges and improve the survey coding experience. For example, SMART will soon migrate to using vector databases for embedding storage, further enhancing label recommendation speed and scalability.
SMART: AI-Driven Survey Coding Solution for Efficient, High-Quality Results
By seamlessly blending cutting-edge AI with mature core coding capabilities, SMART represents a modern solution for eliminating the labor of survey text coding. Its continued evolution—driven by real-world coding use cases—will ensure that efficient, consistent, and high-quality coding remains within reach as the volume and variety of data continually grow.
If you are interested in using SMART, visit our product page to view user documents for more information and to connect with our team of experts.
Learn more about SMART and how RTI continues to innovate with technology.