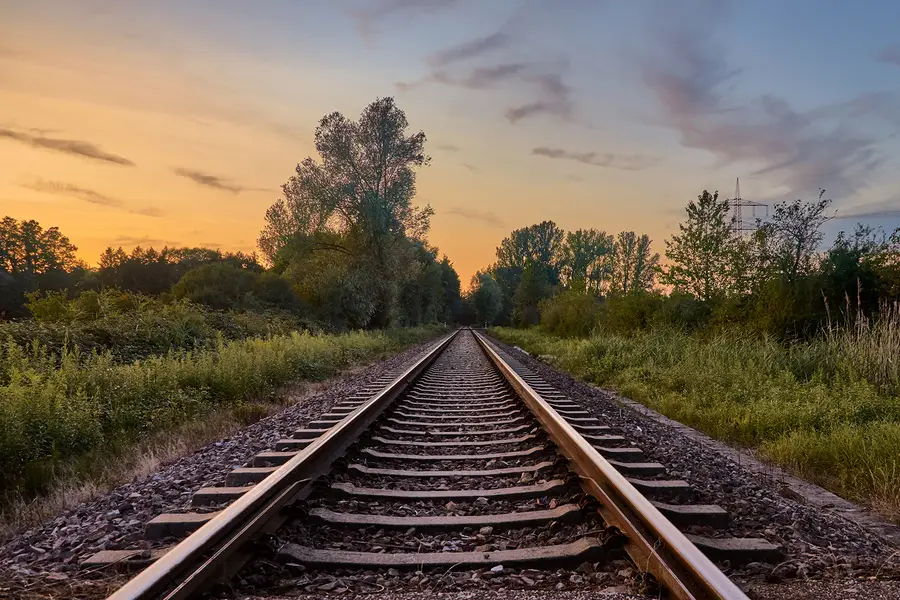
The piece was first published by the Medical Care Blog.
To help determine which health policy changes to the Medicare or Medicaid programs are desirable, the CMS Center for Medicare and Medicaid Innovation (CMMI) relies on formal evaluations, performed by outside contractors, of how smaller scale, typically voluntary, demonstrations and other initiatives impact outcomes of interest. However, determining causal impacts often relies on the key assumption of parallel trends. We can only isolate the treatment impact if the treatment and comparison groups have outcomes evolving in a parallel fashion over time. In this post we report how CMMI evaluations are assessing and addressing parallel or non-parallel trends.
Parallel Trends Matter for Drawing Causal Conclusions
The 2021 Nobel Prize in Economic Sciences recognized the important contribution of econometric methods, developed in the 1990's, that allow researchers to draw causal conclusions from natural experiments. Over the first ten years of CMMI’s operation, these quasi-experimental methods have been used repeatedly. CMMI models have only rarely utilized a randomized controlled trial design. Among quasi-experimental methods, the most frequently used analysis is difference-in-differences (DiD) analysis.
Applying DiD, however, presents a challenge. After identifying a comparison group, one must be able to argue that the comparison serves as a suitable counterfactual. Namely, one must assume that the change measured in the comparison group between the pre-intervention and post-intervention periods approximates what would have occurred in the intervention group, had the intervention not occurred. This is commonly referred to as the “parallel trends assumption”. That is, changes in the intervention and comparison groups would parallel each other except for the impact of the intervention itself.
There is a developing literature (see here and here, for example) on the best approaches to assessing whether the parallel trends assumption holds when applying DiD, and what to do if it does not. Since best practices continue to evolve, evaluators may not always follow the ideal approach. But the ways in which evaluators deal with, or do not deal with, non-parallel trends can affect the results of the evaluation. This is clearly important for evaluations with policy implications.
How Do CMMI Evaluations Assess Parallel Trends?
Assessing parallel trends relies on the observable pre-intervention trends in the baseline period. We can visually inspect pre-intervention trends, but statistical tests are also available. To the extent the pre-intervention trends for the intervention and comparison groups are non-parallel, this serves as evidence against the parallel trends assumption.
In some cases, data availability may drive the decision to test for parallel trends. For example:
- Sample size may not be large enough to detect non-parallel trends (not applicable for most CMMI evaluations).
- Sample size may be so large that tests are always statistically significant, though not practically significant.
- There may not be enough baseline periods of data to assess pre-intervention trends.
To understand how CMMI evaluations assess parallel trends, we reviewed the evaluation reports or Reports to Congress for 51 CMMI models posted between September 2012 and May 2021. We consulted several resources (here, here, and here) to develop the list of CMMI models with formal evaluations. We reviewed the reports to determine if researchers used DiD, whether and how the researchers tested for parallel trends, and the results of the test. The complete list of evaluations reviewed is available in PDF format.
Out of the 51 reports, 40 used DiD. Twenty-three of the evaluations using DiD tested for parallel trends, 19 using statistical tests and four using visual inspection only. As shown in Figure 1, testing for parallel trends was uncommon in CMMI evaluations prior to 2017. Between 2018 and 2019, four out of six reports described tests for parallel trends. Since 2020, 15 out of 17 reports included tests for parallel trends. Only one of the 15 reports used visual inspection only.
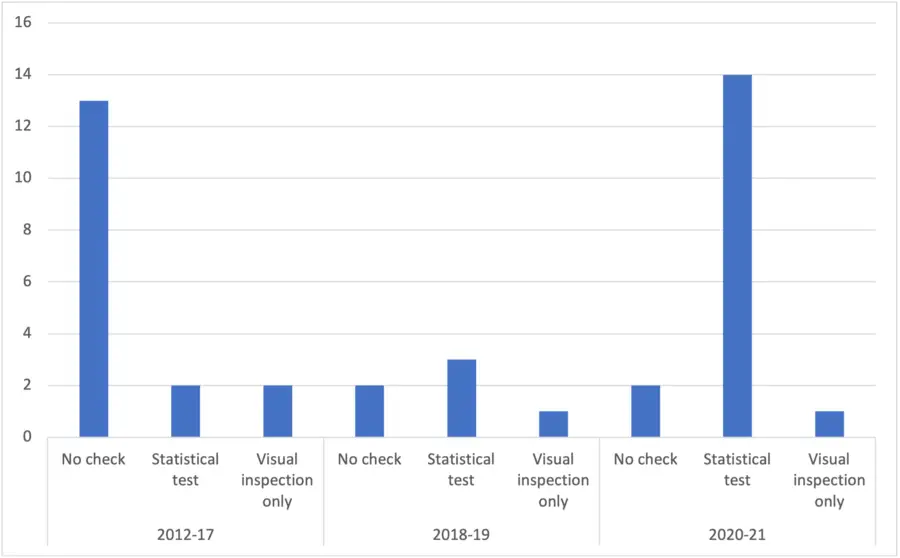
Most CMMI Evaluations Do Nothing to Address Non-Parallel Trends
We also examined the results of the tests for parallel trends and the methodology adjustments used to address non-parallel trends. Of the 19 reports that statistically tested for parallel trends, 15 (79%) found evidence against parallel trends for at least some DiD models. In these cases, evaluators used several approaches:
- Do not make any methodology changes. Most evaluations used a standard DiD estimator that assumes parallel trends even though some outcome variables violated the assumption. The authors either noted, or did not report, the non-parallel outcomes.
- Modify the regression specification. One can adjust the regression specification to net out the expected differential trends. Most adjust the specification by including a linear differential trend term.
- Re-weight the comparison group to ensure parallel trends. There are ways to refine the comparison group methodology to increase the plausibility of parallel trends. For example, you can include outcome trends in the comparison group matching or weighting.
Figure 2 summarizes these options utilized by CMMI evaluations. The most common response by far was to do nothing. Only six evaluations adapted the DiD methodology to address non-parallel trends. Five of these (State Innovation Models Initiative: Round 1, Maryland All-Payer Model, Initiative to Reduce Avoidable Hospitalizations Among Nursing Facility Residents: Phase 2, Home Health Value Based Purchasing Model, and Oncology Care Model evaluations) modified the regression specification. The Medicare Advantage Value-Based Insurance Design Model [MA VBID] re-weighted the comparison group.
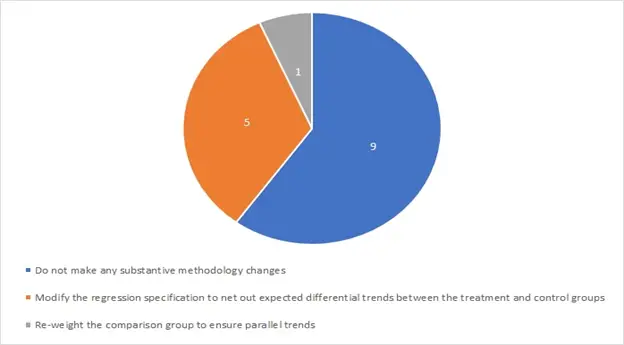
Discussion of Results
The evaluations that modified the regression specification all included a differential linear trend for at least some outcomes. None of these evaluations justified why this approach was better than other methodology adjustments.
The MA VBID evaluation updated their comparison group for each affected outcome to force parallel pre-intervention trends. They used propensity score matching and, when necessary, entropy balancing. It is not unusual to use matching or weighting for the comparison group. But this was the only evaluation to include pre-intervention trends on an outcome-by-outcome basis. Some justification for the approach was provided in an earlier report, but there was no discussion of why it was preferred to a differential linear trend adjustment.
The MA VBID evaluation was the only one to include outcome trends in the comparison group methodology after testing for parallel trends. Other evaluation reports included pre-intervention trends for certain outcomes before testing for parallel trends. Two examples were the Comprehensive Primary Care Plus and Maryland All-Payer Model evaluations.
Testing For Parallel Trends Has Increasingly Become Standard Practice
There is less of a standard practice for what to do when there is evidence against parallel trends. This is usually the case for at least some outcomes (at least in CMMI evaluations). The most common response to non-parallel trends in CMMI evaluations is to not take any action. Most just omitted or flagged results for the affected outcomes.
Doing nothing may be the ideal approach in many circumstances. This includes, for example, when evidence against parallel trends occurs in secondary outcomes or is otherwise practically insignificant. In some cases, however, the evidence against parallel trends could compromise conclusions. There are different ways to adjust the evaluation methodology to address non-parallel trends. A clearer understanding and justification of which adjustments are appropriate in which context could improve policy evaluation.
The authors gratefully acknowledge Olga Khavjou, Rachel Kornbluh, Hannah Wright, Fang He, Marisa Morrison, Heather Beil, and Susan Haber for reviewing evaluation reports and providing helpful suggestions.